Is the choice between a DGX Spark and an A100 simply a matter of performance metrics, or does the decision delve deeper, impacting the very fabric of your organization's computational future? Understanding the nuanced differences between these two powerful platforms is not merely an exercise in comparing specifications; it's a strategic imperative for any entity seeking to harness the transformative potential of accelerated computing.
The landscape of high-performance computing is constantly evolving. For years, NVIDIA has been at the forefront, pushing the boundaries of what's possible with its GPU technology. Within this realm, the DGX systems and the A100 GPUs represent two distinct approaches to achieving unparalleled computational power. The DGX Spark, often representing an earlier iteration or a specific configuration, and the A100, a singular, high-performance GPU architecture, present different trade-offs in terms of scalability, cost, and intended application. The choices businesses make here dictate their ability to innovate, to solve complex problems, and ultimately, to maintain a competitive edge in an increasingly data-driven world. This article will dissect the DGX Spark vs A100, providing a detailed comparison to help you navigate the crucial decision-making process.
Consider the scenario. A research institution wants to accelerate its drug discovery pipelines. A financial firm aims to optimize its algorithmic trading strategies. A large technology company seeks to improve the performance of its deep learning models. In each of these contexts, the question of which computing platform to leverage a DGX Spark or A100-based solutions takes center stage. Understanding the specifications and real-world performance is critical. The choice doesn't hinge solely on raw processing power. It considers factors like ease of deployment, power consumption, support infrastructure, and, crucially, total cost of ownership (TCO). This exploration is not only about the hardware; its also about the software ecosystems, the optimization tools, and the availability of skilled personnel to manage and utilize these complex systems effectively.
Let's consider a general overview to illuminate the distinctions between the two approaches. The DGX systems, in general, are integrated, purpose-built machines that combine multiple GPUs, high-speed interconnects, and optimized software. They are designed to be a complete, ready-to-deploy solution. An A100, conversely, is a single GPU, a building block that can be deployed in various server configurations, from standalone systems to massive, distributed clusters. This modularity offers flexibility but necessitates a more hands-on approach to system integration and management. The choice of which one is better heavily depends on the specific requirements of the end user.
The DGX platform provides an integrated hardware and software experience optimized for ease of use and performance. The A100, while offering exceptional performance in a single card, often requires integration into a broader infrastructure.
Let's delve deeper into the specifics. The DGX system, often incorporating multiple A100 (or earlier generation) GPUs, offers a pre-configured, optimized environment. It streamlines the deployment process by providing a fully integrated solution, minimizing the complexities of configuring hardware and software. This approach can be advantageous for organizations that prioritize rapid deployment and ease of management. In contrast, the A100 is a more versatile, modular component, offering unparalleled raw processing power within a single unit. This flexibility makes it well-suited for building custom, scalable solutions tailored to specific needs.
One of the most important aspects is the architecture. The DGX Spark, as noted, isn't a specific product but represents a class of DGX systems often featuring earlier-generation GPUs. These older GPUs, such as the Tesla V100 or the previous generation of the A100, still offered considerable computational power but were surpassed by newer architectures. The A100, on the other hand, represents a single, cutting-edge GPU featuring the NVIDIA Ampere architecture. The Ampere architecture brought significant advancements in performance, particularly in deep learning workloads, providing substantial gains in training time and inference throughput. The A100 is a marvel of modern engineering, utilizing cutting-edge manufacturing processes and advanced chip design to achieve remarkable processing capabilities.
Beyond the individual components, the interconnect fabric plays a crucial role in the overall system performance. The DGX systems typically leverage NVIDIA's NVLink, a high-speed, direct-GPU-to-GPU interconnect that allows for rapid data transfer between GPUs. NVLink minimizes the latency and maximizes the bandwidth, crucial for large-scale parallel processing tasks such as training deep learning models. A100 GPUs, when integrated into server configurations, also benefit from advanced interconnect technologies, such as NVIDIA's InfiniBand. The choice of interconnect profoundly impacts the scalability and performance of the overall system, especially in multi-GPU configurations, emphasizing the importance of a holistic system perspective.
Software is a crucial part of the equation, and the DGX system generally offers a complete software stack optimized for deep learning, data science, and other accelerated computing tasks. This stack typically includes pre-installed frameworks, libraries, and drivers optimized for NVIDIA GPUs. This streamlines the development process, enabling users to focus on their applications rather than spending time on system setup and configuration. In contrast, users of A100 GPUs often have more flexibility in choosing their software environment, allowing them to tailor the software stack to their specific needs and preferences. This flexibility can be particularly beneficial for organizations with unique software requirements or those who are already invested in a particular development ecosystem.
Power consumption and thermal management are also vital considerations, particularly in data center environments. The DGX systems, with their multi-GPU configurations, generally consume more power than a single A100 GPU. Data center operators need to carefully evaluate the power requirements of their computing infrastructure to ensure that it can meet the demands of these high-performance systems. This involves considering factors such as power distribution, cooling capabilities, and the overall efficiency of the data center infrastructure. The A100, while powerful, is designed to be relatively energy-efficient compared to previous generations, helping to mitigate some of the power and cooling challenges.
Total Cost of Ownership (TCO) is a comprehensive metric that considers all costs associated with owning and operating a computing system, including hardware costs, software licenses, power consumption, cooling, maintenance, and personnel costs. While the initial cost of a DGX system may be higher than that of an A100-based solution, the DGX system's pre-integrated nature and ease of management may result in lower operational costs. This can be especially true for organizations with limited in-house expertise in GPU computing. The choice that works best will also be heavily influenced by how the individual plans to use the solution.
The decision of which platform to select, then, depends heavily on the application. For large-scale deep learning training and inference, the DGX system's integrated environment and optimized software stack can provide a significant advantage, accelerating the development and deployment of complex AI models. However, for applications that require greater flexibility and scalability, such as building custom AI solutions or running a variety of workloads, the A100-based approach may be more suitable. Furthermore, factors such as budget constraints, available technical expertise, and long-term growth plans play critical roles in the decision-making process. The best choice will be the one that is in the best interests of the company.
The software side is critical to the success of any GPU deployment. The DGX systems come with NVIDIA's Deep Learning Software Stack pre-installed. This stack includes optimized versions of popular deep learning frameworks like TensorFlow, PyTorch, and others, as well as NVIDIA's own libraries and tools. This pre-optimized environment reduces the time and effort required to configure and optimize the system, allowing users to focus on developing and deploying their AI applications. For A100 users, while they have the flexibility to customize their software stack, they are also responsible for the setup, configuration, and optimization of the frameworks, libraries, and drivers. While the user has complete control over the installation, there is additional work that must be done to get the system ready for use.
The flexibility of the A100-based approach is also an attractive aspect. A100 GPUs can be deployed in a variety of server configurations, ranging from single-GPU workstations to large-scale, multi-GPU clusters. This modularity allows organizations to scale their computing resources as their needs evolve. It offers the flexibility to purchase and deploy individual GPUs as needed, making the investment more scalable and adaptable to changing workloads. DGX systems, by contrast, are a more integrated solution and are less easily adapted.
Consider also the concept of future-proofing. NVIDIA, as a leader in GPU technology, continually releases new generations of GPUs. Choosing the right platform can help ensure that your investment remains current and that you can take advantage of the latest technological advancements. The A100, being a single GPU, provides the flexibility to upgrade individual components as newer generations become available. This approach helps to mitigate the risk of obsolescence and ensures access to the latest performance and efficiency gains. The DGX systems can be upgraded too, but often require a more significant investment to replace the entire system.
Ultimately, the decision between a DGX Spark and an A100 is a nuanced one. There is no one-size-fits-all answer. The best choice depends on the specific needs of the organization, including budget constraints, the types of workloads that will be run, and the availability of in-house expertise. A DGX Spark might be a more accessible option and is easier to deploy for teams seeking a complete, pre-configured solution. The A100, on the other hand, provides greater flexibility, scalability, and cost-effectiveness for organizations with the technical resources to build and manage their systems. A careful assessment of the organization's requirements is essential before making a final decision.
Lets analyze the practical implications of choosing between a DGX Spark and an A100, consider a specific example. A team focused on natural language processing (NLP) research. They're working on training large language models (LLMs) with billions of parameters. Training these models requires a system with significant computational power and memory. They have two options: Option 1: Invest in a DGX Spark system. Option 2: Purchase several A100 GPUs and build their own custom server cluster. The DGX Spark offers an advantage in this scenario because of its integrated hardware and software stack. NVIDIA's optimized software helps reduce the time and effort required to set up and configure the system. This allows the team to focus on model development and experimentation. The pre-configured and optimized environment can be particularly beneficial, as the NLP team might lack the in-house expertise to manage a complex GPU cluster.
On the other hand, if the NLP team has strong in-house expertise in system administration and is looking to maximize their investment, they might choose the A100-based approach. By building their own server cluster, they can scale their computing resources more granularly, adding more GPUs as needed. This flexibility helps them to adapt to changing workloads and evolving research requirements. Moreover, they might be able to save money on the initial investment. The custom cluster approach also gives them greater control over the hardware configuration and software stack, allowing them to tailor the system to their specific needs.
The decision for the team should consider various factors. The DGX Spark's ease of deployment and management can result in faster time-to-value. The A100 approach offers a higher degree of customization, scalability, and potentially lower initial cost. For the NLP team, the choice depends on their budget, technical expertise, and long-term research goals. The DGX Spark simplifies the set-up, and the team can get started quickly. However, the A100 cluster provides the most scalable solution as the team continues to conduct more research.
Another consideration is the concept of vendor lock-in. DGX systems represent a more integrated solution, which can lead to increased reliance on a single vendor. While NVIDIA is a leader in GPU technology, organizations might want to avoid being locked into a specific vendor. An A100-based solution provides greater flexibility, allowing organizations to choose hardware and software components from different vendors. This flexibility can be crucial for organizations that prefer to have more control over their technology stack.
The DGX Spark systems are easier to deploy and manage. But the A100 offers greater flexibility, scalability, and potentially lower costs for organizations with the necessary technical expertise.

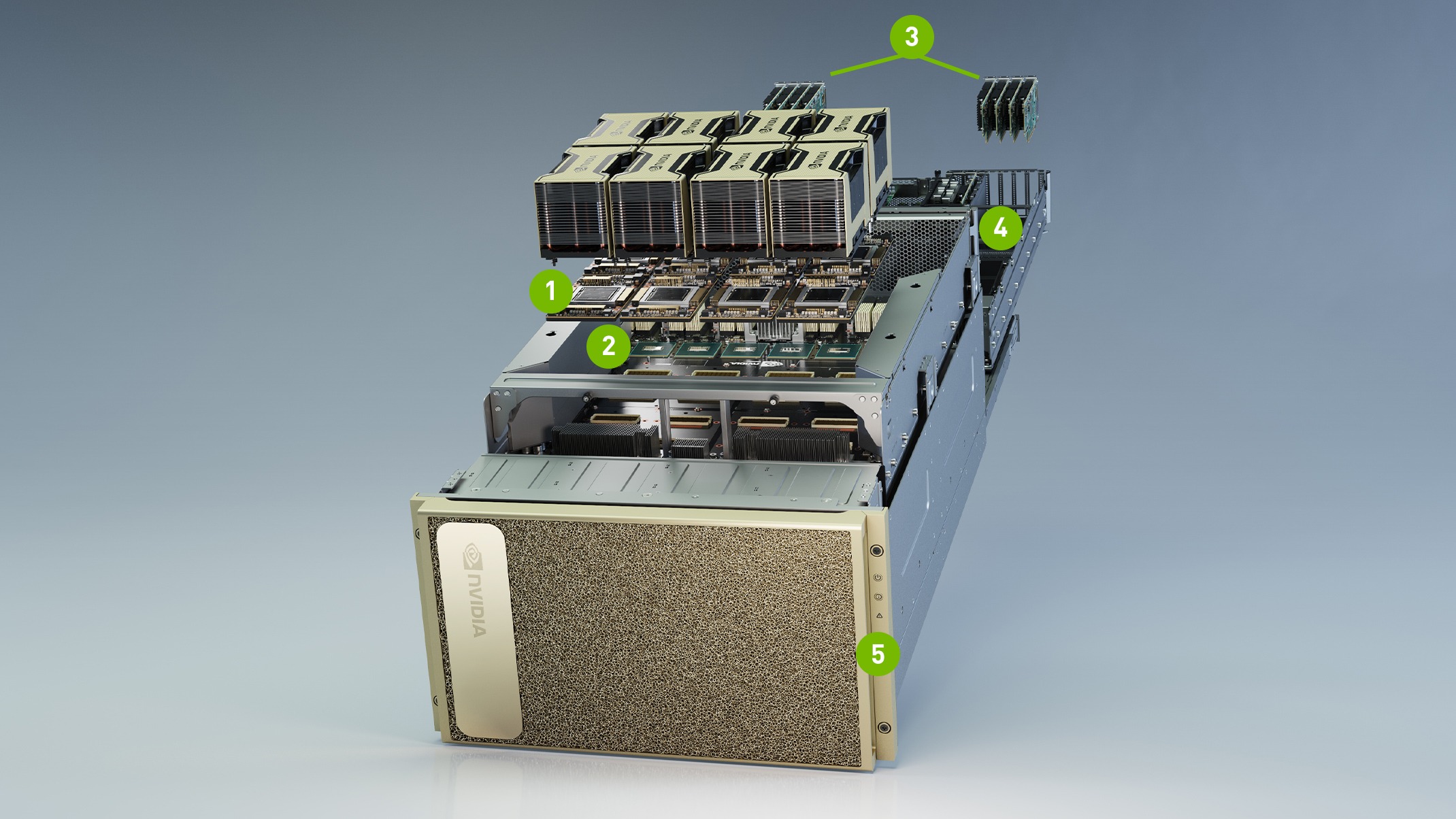
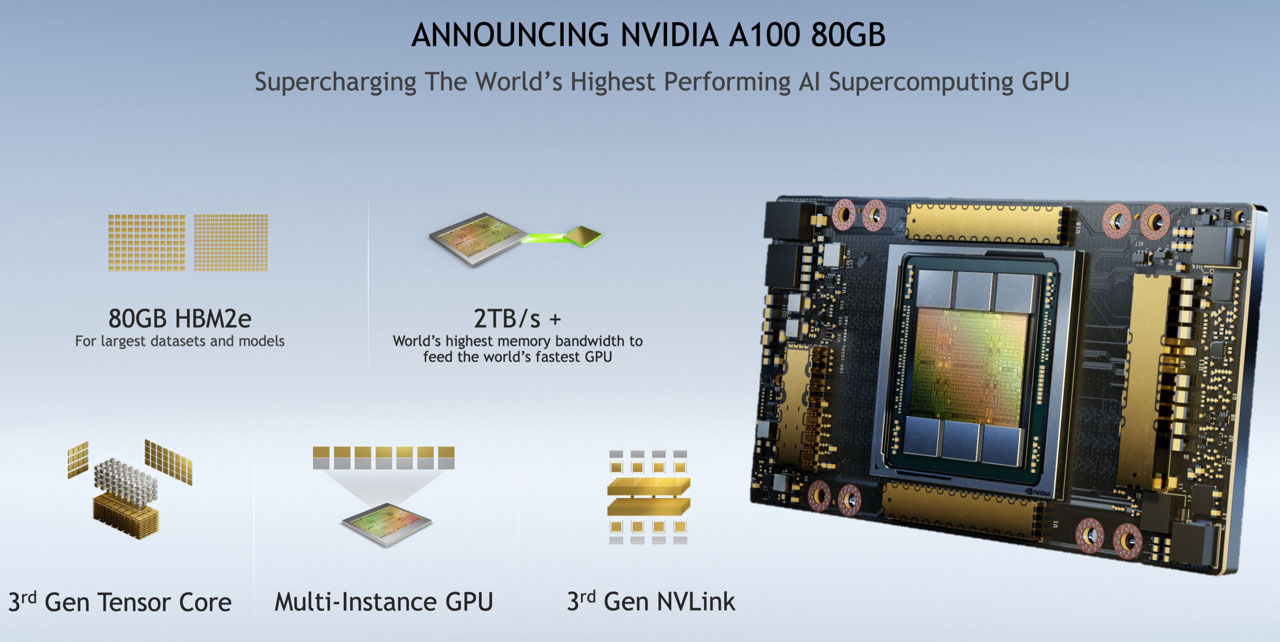